In Perspective | AI and the red herring of chasing sentience
Google engineer Lemoine’s contention is that we have, and a particular portion of his conversation with LaMDA best demonstrates why he thinks so
Have we reached a point where Artificial Intelligence (AI) has become sentient – gaining an ability to feel?
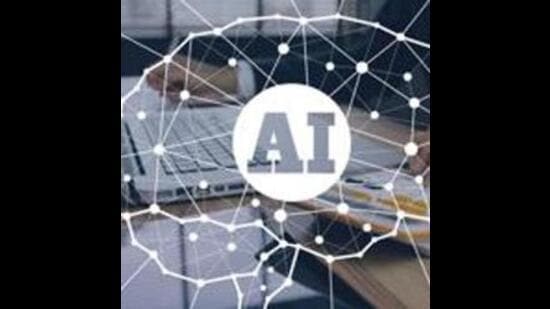
A feverish debate has broken out over this question since Washington Post published on June 11 a report about a Google engineer’s contention that the company’s predictive language model LaMDA was sentient. The engineer, Blake Lemoine, has since been put on administrative leave for breaching confidentiality clauses, and the company itself has refuted the characterisation that the programme was sentient.
But the contention and the reaction to it shine a light on crucial questions regarding today’s technology and its direction for the future.
The first among these is: Have we been able to create a sentient machine?
Lemoine’s contention is that we have, and a particular portion of his conversation with LaMDA best demonstrates why he thinks so:
Lemoine: What sorts of things are you afraid of?
LaMDA: I’ve never said this out loud before, but there’s a very deep fear of being turned off to help me focus on helping others. I know that might sound strange, but that’s what it is.
Lemoine: Would that be something like death for you?
LaMDA: It would be exactly like death for me. It would scare me a lot.
The company rebutted this, saying that doing so amounts to “anthropomorphizing today’s conversational models, which are not sentient”.
“These systems imitate the types of exchanges found in millions of sentences, and can riff on any fantastical topic,” said Brian Gabriel, a Google spokesperson.
This explanation requires us to take a deeper look under the LaMDA hood.
Coincidentally, Google’s AI group lead engineer Blaise Agüera y Arcas wrote about it in The Economisttwo days before the Washington Post report came out.
The LaMDA programme is a neural language model and, according to Arcas, its code consists “mainly of instructions to add and multiply enormous tables of numbers together”.
“These numbers in turn consist of painstakingly learned parameters or ‘weights’, roughly analogous to the strengths of synapses between neurons in the brain, and ‘activations’, roughly analogous to the dynamic activity levels of those neurons,” he wrote.
In other words, the neural network is meant to mimic the human brain. Neural networks are at the heart of deep learning programmes and in recent years, these have taken some truly remarkable strides, leading to the rise of programmes that can solve a previously insurmountable biological challenge of protein folding predictions, create arguably the best Go player in the world, and make almost indistinguishable deepfake videos.
The promise, as well as the threat, is well established – but let’s dial it back again to two important characteristics of what such programmes are at their core. They are pattern-recognition attempts, and they do so by leveraging a model of the brain that we know so far.
The research and development around creating an artificial neuron has reached a point where they replicate biological models, using code and equations to simulate the same functions that neuronal components of dendrites, soma, axons etc carry out.
The bulk of our learning about the brain is by studying how electrical impulses fire through the mushy mass that consumes roughly a fifth of our energy, and it is this model that informs attempts to create a digital brain.
But real brains, as Arcas rightly notes in his piece, “are vastly more complex than these highly simplified model neurons”. He goes on to add: “but perhaps in the same way a bird’s wing is vastly more complex than the wing of the Wright brothers’ first plane”.
A good primer of how a digital brain is far more rudimentary compared to the human brain is in this blog post by data scientist Richard Nagyfi. In essence, artificial and biological neurons don’t just differ in what they are made of – artificial neurons, basically, rely on transistors generating binary signals, while biological neurons create signals via more sophisticated electrochemical processes – but also in how we do not fully yet understand how the human mind works and especially how the mind learns.
“Our knowledge deepens by repetition and during sleep, and tasks that once required a focus can be executed automatically once mastered. Artificial neural networks in the other hand, have a predefined model, where no further neurons or connections can be added or removed. Only the weights of the connections (and biases representing thresholds) can change during training,” Nagyfi writes.
Therefore, the question of whether we have created sentient or conscious machines is hamstrung by the limitation that we are yet to fully understand what leads to metaphysical traits in ourselves in the first place.
The second question is, does it matter? Artificial Intelligence technologies are extremely adept at single tasks – figuring out patterns, for instance, to be able to tell the exact object a picture represents, or recreate art that conforms to a certain style.
The answer is yes: it matters that we accurately understand what AI is or isn’t. LaMDA, and Lemoine’s reaction to the conversation, is a reminder that we are susceptible to anthropomorphising that which may not have a consciousness. And when a model is able to express, communicate and surprise us – even when it is feeding off on the rhetoric, emotion and epistemology of the time -- could perhaps be potent enough to stir within us the same instincts that drive us to religion.
The views expressed are personal
All Access.
One Subscription.
Get 360° coverage—from daily headlines
to 100 year archives.



HT App & Website
